## Meta-Album DIBaS Dataset (Micro)
*
The Digital Images of Bacteria Species dataset (DIBaS) (https://github.com/gallardorafael/DIBaS-Dataset) is a dataset of 33 bacterial species with around 20 images for each species. For the Meta-Album, since the images were large (2 048x1 532) with very few samples in each class, we decided to split each image into several smaller images before resizing them to 128x128. We then obtained a preprocessed dataset of 4 060 images with at least 108 images for each class. This dataset was also preprocessed with blob normalization techniques, which is quite unusual for this type of image. The goal of this transformation was to reduce the importance of color in decision-making for a bias-aware challenge.
### Dataset Details
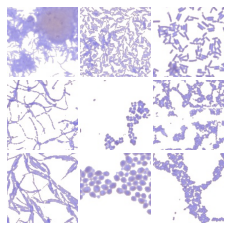
Meta Album ID: MCR.BCT
Meta Album URL: [https://meta-album.github.io/datasets/BCT.html](https://meta-album.github.io/datasets/BCT.html)
Domain ID: MCR
Domain Name: Microscopic
Dataset ID: BCT
Dataset Name: DIBaS
Short Description: Digital Image of Bacterial Species (DIBaS)
\# Classes: 20
\# Images: 800
Keywords: microscopic, bacteria
Data Format: images
Image size: 128x128
License (original data release): Public for researchers
License URL(original data release):
License (Meta-Album data release): CC BY-NC 4.0
License URL (Meta-Album data release): [https://creativecommons.org/licenses/by-nc/4.0/](https://creativecommons.org/licenses/by-nc/4.0/)
Source: Digital Image of Bacterial Species (DIBaS)
Source URL: http://misztal.edu.pl/software/databases/dibas/
https://journals.plos.org/plosone/article?id=10.1371/journal.pone.0184554
https://github.com/gallardorafael/DIBaS-Dataset
Original Author: Bartosz Zielinski, Anna Plichta, Krzysztof Misztal, Przemyslaw Spurek, Monika Brzychczy-Wloch, Dorota Ochonska
Original contact: krzysztof.misztal@uj.edu.pl
Meta Album author: Romain Mussard
Created Date: 01 March 2022
Contact Name: Ihsan Ullah
Contact Email: meta-album@chalearn.org
Contact URL: [https://meta-album.github.io/](https://meta-album.github.io/)
### Cite this dataset
```
@article{10.1371/journal.pone.0184554,
doi = {10.1371/journal.pone.0184554},
author = {Zielinski, Bartosz AND Plichta, Anna AND Misztal, Krzysztof AND Spurek, Przemyslaw AND Brzychczy-Wloch, Monika AND Ochonska, Dorota},
journal = {PLOS ONE},
publisher = {Public Library of Science},
title = {Deep learning approach to bacterial colony classification},
year = {2017},
month = {09},
volume = {12},
url = {https://doi.org/10.1371/journal.pone.0184554},
pages = {1-14},
number = {9}
}
```
### Cite Meta-Album
```
@inproceedings{meta-album-2022,
title={Meta-Album: Multi-domain Meta-Dataset for Few-Shot Image Classification},
author={Ullah, Ihsan and Carrion, Dustin and Escalera, Sergio and Guyon, Isabelle M and Huisman, Mike and Mohr, Felix and van Rijn, Jan N and Sun, Haozhe and Vanschoren, Joaquin and Vu, Phan Anh},
booktitle={Thirty-sixth Conference on Neural Information Processing Systems Datasets and Benchmarks Track},
url = {https://meta-album.github.io/},
year = {2022}
}
```
### More
For more information on the Meta-Album dataset, please see the [[NeurIPS 2022 paper]](https://meta-album.github.io/paper/Meta-Album.pdf)
For details on the dataset preprocessing, please see the [[supplementary materials]](https://openreview.net/attachment?id=70_Wx-dON3q&name=supplementary_material)
Supporting code can be found on our [[GitHub repo]](https://github.com/ihsaan-ullah/meta-album)
Meta-Album on Papers with Code [[Meta-Album]](https://paperswithcode.com/dataset/meta-album)
### Other versions of this dataset
[[Mini]](https://www.openml.org/d/44281) [[Extended]](https://www.openml.org/d/44316)